南开大学旅游学系素来秉承与弘扬“允公允能、日新月异”的校训,不断关注科技发展前沿,紧跟时代步伐,致力于推动中国特色旅游理论体系、学科体系和话语体系的构建,为新发展格局下旅游业高质量发展注入了强大动力。以我院百名青年学科带头人培养计划入选者毕建武副教授为代表的科研团队,致力于推进旅游大数据深度分析与智能决策研究。该团队通过利用最先进的大数据分析技术和人工智能技术,对旅游大数据进行深入分析与挖掘,开发有效的预测与决策支持模型,旨在推动旅游业实现智能化、数字化和信息化的发展。
毕建武博士毕业于东北大学,目前为旅游与服务学院副教授、博士生导师,入选南开大学百名青年学科带头人培养计划,担任中国优选法统筹法与经济数学研究会智能决策与博弈分会、中国管理现代化研究会管理与决策科学专业委员会等理事;主持2项国家级科研项目和多项省部级科研项目,并以核心成员参与国家自然科学基金、国家社会科学基金、教育部人文社科基金近10项。近5年在《Tourism Management》、《Annals of Tourism Research》、《Journal of Travel Research》、《International Journal of Contemporary Hospitality Management》、《International Journal of Production Research》、《Information Sciences》、《Information Fusion》、《Tourism Economics》等国内外旅游学科和关联学科的重要期刊发表高质量研究成果30余篇;发表的研究已经引起了国内外学者的广泛关注,目前google学术引用近1500次,其中2篇研究成果入选ESI热点论文名单,3篇研究成果入选ESI高被引论文名单。
长期以来,毕建武博士带领学术团队重点聚焦于旅游大数据与决策分析方向的研究,在旅游大数据深度分析与智能决策领域进行了广泛而深入的探索,取得了丰硕的研究成果,为推进南开旅游学系大数据智能挖掘篇章作出了重要贡献。具体研究工作包括:
1. 提出基于在线评论的旅游与服务产品改进设计方法
随着信息技术的迅猛发展,在电商网站、OTA等平台上可以看到针对各类产品的在线评论信息大量涌现。这些在线评论中含有丰富的有价值信息,可以支持旅游与服务产品的改进设计。如何基于海量的在线评论信息分析对旅游与服务产品进行改进设计,这是具有重要理论意义及实际应用价值的研究课题。为此,团队在国际上较早且系统性地开展了基于在线评论的产品改进设计方法研究,提出了一系列分析方法与技术。与传统的采用调查问卷的方法相比,基于在线评论数据的分析方法能够节约大量的时间和经济成本,为旅游与服务产品的改进完善提供实时、科学的建议支持。近5年以来,围绕该方面研究,毕建武博士以第一作者或通讯作者发表SCI/SSCI检索论文7篇,发表的论文被引用400余次,入选ESI高被引论文2篇。具有代表性的研究成果发表在国际重要学术期刊《Tourism Management》、《Annals of Tourism Research》、《International Journal of Production Research》等期刊上,并得到营销领域顶级期刊《Marketing Science》前主编耶鲁大学K Sudhir教授、著名国际期刊《Electronic Commerce Research and Applications》高级主编美国弗吉尼亚理工大学Alan Wang教授、著名国际期刊《IISE Transactions Design and Manufacturing》领域主编伊利诺伊大学香槟分校Harrison Kim教授、国际著名期刊《Tourism Management》、《Tourism Review》等编委会成员韩国世宗大学Heesup Han教授、国际著名期刊《Tourism Management》、《Journal of Business Research》等编委会成员雷丁大学Marcello Mariani教授、湖南大学张跃军教授等、四川大学徐泽水教授等人的直接引用和正面评价。
2. 提出基于多源异构数据的旅游需求预测理论与方法
随着信息技术的发展,出现了多种数据可以辅助旅游需求预测。因此,如何基于多源数据对旅游需求进行精确预测,这是具有理论意义及实际应用价值的研究课题。为此,团队在国际上较早且系统性地开展了大数据背景下的旅游需求预测研究,提出了基于多源数据的旅游需求深度学习预测方法、基于大数据的旅游需求集成深度学习预测方法、基于旅游需求时序数据图像编码的深度学习预测方法。团队提出的理论与方法能够有效的提高旅游需求预测精度,为政府及旅游相关企业制定战略发展、投资决策、收益管理、定价策略等提供科学依据。围绕该方面研究,毕建武博士以第一作者或通讯作者发表学术论文8篇,代表性研究成果发表在旅游管理国际顶级期刊《Annals of Tourism Research》和《Journal of Travel Research》中,并得到ATR副主编弗吉尼亚理工大学Nicolau教授、美国天普大学Yang教授、发展中国家院士汪寿阳教授等人的直接引用和正面评价。
3. 提出基于在线评论的旅游产品排序推荐方法
随着web 2.0时代的到来,越来越多的人通过互联网购买或预定产品。与实体店购物不同,通过互联网购物通常无法预先体验产品。消费者通常会通过阅读在线评论来增进对产品的了解,然而在线评论数量众多,消费者很难逐条的阅读评论并做出决策。如何依据在线评论确定产品排序,对于支持消费者决策具有重要意义。为此,团队在国际上较早且系统性地开展了基于在线评论的旅游产品排序推荐方法研究,提出了一系列排序推荐方法,对于减少消费者搜索时间、促进购买具有重要意义。围绕该方面研究,毕建武博士以第一作者或通讯作者发表学术论文8篇,被引用500余次。具有代表性的研究成果发表在国际重要学术期刊《Annals of Tourism Research》,《Information Fusion》、《Information Sciences》、《Expert Systems with Applications》等期刊上,并得到国际期刊《Information Sciences》主编、加拿大科学院院士Pedrycz教授、国际期刊《Information Fusion》主编西班牙格拉纳达大学Herrera教授、国际期刊《Knowledge-Based Systems》前主编日本岩手大学Fujita教授、美国爱荷华州立大学Tang教授的直接引用和正面评价。
作为我国旅游学科领域的先行者,南开大学旅游与服务学院自创建以来,积极主动地肩负旅游学科发展的时代重担,追逐新时代发展的核心命题。依托大数据背景,响应大数据驱动的管理与决策研究国家重大科研需求和科技发展趋势,契合数字经济的发展国家战略,深耕旅游大数据深度分析与智能决策研究,推动旅游业智能化、数字化和信息化的发展。
近期的部分代表性成果如下:
1. 毕建武,2023,“服务属性分类与服务要素配置——基于在线评论情感分析的方法”,中国社会科学出版社 (该专著得到国家社科基金资助于2023年4月正式出版)
2. Bi, J. W., Liu, Y., Fan, Z. P., & Zhang, J. (2019). Wisdom of crowds: Conducting importance-performance analysis (IPA) through online reviews. Tourism Management, 70, 460-478. (ESI 高被引和热点论文)
3. Bi, J. W., Liu, Y., & Li, H. (2020). Daily tourism volume forecasting for tourist attractions. Annals of Tourism Research, 83, 102923.
4. Bi, J. W., Li C., Xu H. & Li, H. (2022). Forecasting daily tourism demand with big data: An ensemble deep learning method. Journal of Travel Research, 61(8), 1719-1737.
5. Bi, J. W., Liu, Y., Fan, Z. P., & Zhang, J. (2020). Exploring asymmetric effects of attribute performance on customer satisfaction in the hotel industry. Tourism management, 77, 104006. (ESI 高被引论文)
6. Bi, J. W., Li, H., & Fan, Z. P. (2021). Tourism demand forecasting with time series imaging: A deep learning model. Annals of Tourism Research, 90, 103255.
7. Gao, G. X., & Bi, J. W.* (2021). Hotel booking through online travel agency: Optimal Stackelberg strategies under customer-centric payment service. Annals of Tourism Research, 86, 103074.
8. Bi, J. W., Liu, Y., Fan, Z. P., & Cambria, E. (2019). Modelling customer satisfaction from online reviews using ensemble neural network and effect-based Kano model. International Journal of Production Research, 57(22), 7068-7088.
9. Chen, D., & Bi, J.W.* (2022). Cue congruence effects of attribute performance and hosts’ service quality attributes on room sales on peer-to-peer accommodation platforms. International Journal of Contemporary Hospitality Management, 34(10), 3634-3654.
10. Bi, J. W., Han, T. Y., & Yao, Y. (2023). Fine-grained tourism demand forecasting: A decomposition ensemble deep learning model. Tourism Economics.
11. Bi, J. W., Han, T. Y., & Yao, Y. (2023). Collaborative forecasting of tourism demand for multiple tourist attractions with spatial dependence: A combined deep learning model. Tourism Economics.
12. Bi, J. W., Liu, Y., & Fan, Z. P. (2019). Representing sentiment analysis results of online reviews using interval type-2 fuzzy numbers and its application to product ranking. Information Sciences, 504, 293-307.
13. Bi, J. W., Liu, Y., & Fan, Z. P. (2020). Crowd intelligence: Conducting asymmetric impact-performance analysis based on online reviews. IEEE Intelligent Systems, 35(2), 92-98.
14. Liu, Y., Bi, J. W.*, & Fan, Z. P. (2017). Multi-class sentiment classification: The experimental comparisons of feature selection and machine learning algorithms. Expert Systems with Applications, 80, 323-339.
15. Bi, J.W., Han, T. Y., Yao, Y., & Li, H. (2022). Ranking hotels through multi-dimensional hotel information: a method considering travelers’ preferences and expectations. Information Technology & Tourism, 24(1), 127-155.
16. Bi, J. W., Han, T. Y., & Li, H. (2020). International tourism demand forecasting with machine learning models: The power of the number of lagged inputs. Tourism Economics, 28(3), 621-645.
17. Cheng, H., Liu, Q., & Bi, J. W.* (2021). Perceived crowding and festival experience: The moderating effect of visitor-to-visitor interaction. Tourism Management Perspectives, 40, 100888.
18. Liu, Y., Bi, J. W., & Fan, Z. P. (2017). Ranking products through online reviews: A method based on sentiment analysis technique and intuitionistic fuzzy set theory. Information Fusion, 36, 149-161. (ESI 高被引论文)
19. Liu, Y., Bi, J. W., & Fan, Z. P. (2017). A method for multi-class sentiment classification based on an improved one-vs-one (OVO) strategy and the support vector machine (SVM) algorithm. Information Sciences, 394, 38-52.
20. Liu, Y., Bi, J. W.*, & Fan, Z. P. (2017). A method for ranking products through online reviews based on sentiment classification and interval-valued intuitionistic fuzzy TOPSIS. International Journal of Information Technology & Decision Making, 16(06), 1497-1522.
21. Bi, J. W., Liu, Y., & Fan, Z. P. (2020). A deep neural networks based recommendation algorithm using user and item basic data. International Journal of Machine Learning and Cybernetics, 11(4), 763-777.
22. He, L. Y., Li, H.*, Bi, J.W., Yang, J. J., & Zhou, Q. (2022). The impact of public health emergencies on hotel demand-Estimation from a new foresight perspective on the COVID-19. Annals of Tourism Research, 94, 103402.
23. Chang, J. L., Li, H.*, & Bi, J.W. (2022). Personalized travel recommendation: a hybrid method with collaborative filtering and social network analysis. Current Issues in Tourism, 25(14), 2338-2356.
24. 毕建武, 刘洋, 樊治平 (2018). 依据在线评论的商品排序方法.系统工程学报, 33(03):422-432. (国家自然科学基金委管理科学部认定A级重要期刊)
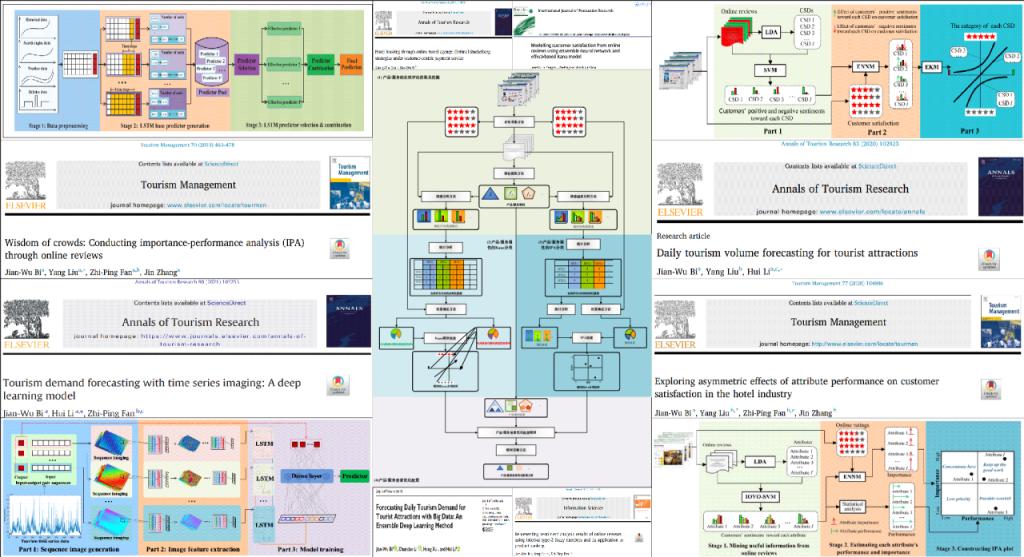